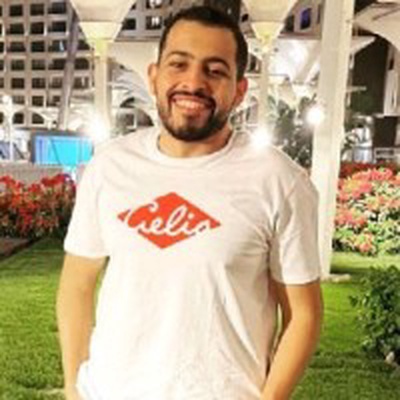
Mohamed E.
About Me
Mohamed is a Data Scientist providing expertise on the full project lifecycle of data solutions from analytic problem definition through data gathering, analysis, model development, reporting/visualization development, testing, and operational deployment. He applies deep learning and computer vision techniques in building models, performing statistical analysis and creating visualizations to provide scalable solutions on projects.